Sustainable Data Governance for Marketers

How can good data governance help you better manage your organization’s marketing metrics? Learn how to prioritize responsible data practices, enhance digital sustainability, and drive continuous learning and improvement with this ten-point data governance plan.
As an agency, one of the most consistent challenges we run into with clients is how they manage their marketing data. The organizations we work with run the data governance gamut:
- Data-impaired: Data is not a strategic priority nor is it used in organizational decision-making. Plus, teams often don’t have analytical skills needed to successfully prioritize good data management.
- Localized: A few key people have data skills but the organization overall doesn’t understand its value or how to effectively manage data.
- Aspirational: Data processes become more standardized and organizational leadership is learning to use data to drive decision-making.
- Analytical: Leadership is engaged and prioritizes data and analytics through team skills-building, investments in business intelligence tools, and so on.
- Strategic: The entire organization is committed to achieving better outcomes with systems in place to build capacity and provide equitable access to data, analytic tools, and training.
Unfortunately, many of the organizations we interact with fall into the first few categories above. Data is strewn across multiple third-party systems in their digital supply chain. Plus, there are few, if any, digital governance programs in place to help teams build capacity, dispose of unused or outdated data, or mitigate unintended consequences associated with digital product, service, or campaign data.
This isn’t unique to marketing either. Departments across organizations struggle to find impactful data governance practices that are effective, viable, and manageable over time. In fact, most organizations rely on third-party providers to manage their data in order to ease this burden. However, this often comes at a significant cost, especially when those providers sell your data to others.
The Consequences of Poor Data Governance
We store all this crap data because it costs less to store it than it costs to think about how to clean it up. We also store it because there is a culture in technology that doesn’t think very deeply about the quality of data. To the average technologist, data is a storage problem, not a knowledge opportunity.
— Gerry McGovern, Getting Rid of Crap Data
It doesn’t take long before a lack of functional data policies and practices cause problems that cost organizations time, money, and resources. Plus, in addition to using valuable resources that could serve organizations elsewhere, the social and ecological ramifications of our collective love affair with all things data-related are significant as well:
- Data commoditization: Figures vary depending on who you ask, but an average internet user produces about 146,880 MB of data per day. By 2025, global data creation is expected to grow to more than 180 zettabytes. What’s more, we’ve turned data into a commodity used to drive inequality and huge profits for some of tech’s biggest players.
- Data privacy & security: Governments around the world work on legislative guidance to keep the data privacy crisis caused by Surveillance Capitalism in check. Yet, to date, these laws haven’t made progress at a pace needed for necessary change to occur. Plus, with this track record, emerging greenwashing and climate disclosure regulations could follow a similar path.
- Data pollution: Also, about two-thirds of organizational data never gets used, which turns power-hungry data centers into the virtual equivalent of landfills.
- Data center growth: Coupled with the rise of AI, blockchain, and other emerging technologies, poor data governance also contributes to a rapid rise in data center construction, which is predicted to double by 2030. These centers disrupt communities and use massive amounts of energy and water.
What’s more, poor data governance can also leave behind a trail of technical debt and employee attrition, among other things. More importantly, it undermines long-term digital resilience, which puts an organization at risk and can produce even more problematic circumstances.
Data and Big Tech Business Models
The reality is that Big Tech companies—where we collect and store most of our data—want you to create more data, not less. They thrive on our lack of data governance to drive their extractivist practices. Their business models depend on it. However, as we can see, this causes major problems.
If we want to redefine digital marketing success to address these issues, we need to redesign our collective relationship with data. Here’s how a conscientious organization can use good data governance practices to better manage assets and processes over time.
Understanding Data Governance
Data governance is a system for defining who within an organization has authority and control over data assets and how those data assets may be used. It encompasses the people, processes, and technologies required to manage and protect data assets.
— What is data governance? Best practices for managing data assets, CIO
We cover governance a lot on this blog, mostly because it is so critical to our clients’ success yet so few do it effectively. Poor governance represents one of the biggest gaps we see in our clients’ operations.
Plus, as a Certified B Corp, effective governance is key to how we create and nurture our own success. Stakeholder governance is a primary driver of the B Corp movement.
Whether it’s content governance, overall digital governance, or specific to marketing data as in this post, good governance helps organizations drive policies and make more informed business decisions that create meaningful change over time.
Data governance success within an organization requires two distinct elements:
- Data governance: The policies and documentation that define ownership, access, sources, and regulatory compliance of an organization’s data.
- Data management: The methods by which an organization executes its data governance policies over time.
Organizations of all stripes need both of these things for success to ensure their data is secure, private, equitable, accurate, and usable. The ten–point plan below outlines how to do this.
Ten Steps to Better Data Governance
The steps in this plan will improve your team’s data governance practices and drive long-term continuous improvement. Once you go through this process, be sure to consistently revisit your efforts to balance efficiency and resource conservation with specific organizational goals.
1. Start with a Sustainable Data Strategy
You can’t prove in advance that your strategy will succeed. You can look at a plan and say all these things are doable. Let’s just do those because they’re within our control. But they won’t add up to much. In strategy, you have to say, if our theory is right about what we can do and how the market will react, this will position us in an excellent way. Just accept the fact that you can’t be perfect on that, and you can’t know for sure.
— Roger Martin, A Plan is Not a Strategy, Harvard Business Review (YouTube video)
To create a flexible and more sustainable data strategy, first answer the following questions:
- How will you define success?
- What capabilities do you need to achieve that success?
- How will you manage and resource strategies over time so that outcomes are prioritized over outputs?
Also consider the following:
- Stakeholder feedback: Use stakeholder mapping to understand key player needs that inform your strategy. Who will be affected by your decisions?
- Workshop your strategy: A good creative collaboration workshop remains one of the best ways to define success and help your team understand how to achieve it.
- Stay lean: If possible, define your strategy in one page or less and be prepared to pivot if something isn’t working (see point #4 below).
- Continuous improvement: To positively impact sustainability, data strategy must be driven by a culture of continuous learning and improvement, but not so much that it drives attrition and gives people implementation whiplash.
Plus, by applying sustainability principles to responsible data governance practices, you’ll create efficient data programs where ethics and responsibility drive better organizational business decisions over time.
2. Data-Driven Decision-Making
Next, it takes time, effective policies, training, and regular reinforcement for teams to acclimate to changing practices. To support data as a crucial part of day-to-day organizational decision-making processes, consider the following:
- Experimentation: Treat business problems as hypotheses to be tested. Create and measure ongoing experiments that move you closer toward your goals.
- Embrace failure: Learning from failure is a natural byproduct of experimentation. However, traditional businesses don’t often support a culture that embraces failure as a natural part of learning and problem-solving. Encourage team members to share what they learn from failures as well as successes.
- Define KPIs: Focus on the metrics that matter most to reach your goals. It can be tempting to measure All The Things. Bloated analytics tools only complicate this issue. However, tracking too many data points—especially misleading ‘vanity’ metrics that don’t help you make clear goal progress—is overkill. In addition to providing a false sense of security, this wastes valuable time and resources.
3. Data-Driven Research and Analysis
What does the data say? If your team can’t answer this question at every step of a project or initiative, perhaps they’re data-impaired. Incorporate ongoing testing into your team and workplace culture. Use this to drive questions and data insights at all levels of the organization and for every step of a project or initiative.
- Resource allocation: Allocate enough time and resources to set your team(s) up for success. Research and analysis are often the first to go when timelines or budgets are tight. Plan accordingly to prioritize these things.
- Clear processes: Create clear research and analysis processes so team members understand how to get the insights they need for long-term success.
- Automation: Incorporate responsible automation tools and processes to improve efficiency. However, use these tools wisely, not as a crutch to mask a lack of skills or data gaps.
- Team training: Most importantly, use some of the allocated resources mentioned above to build capacity and level-up your team’s data research and analysis skills.
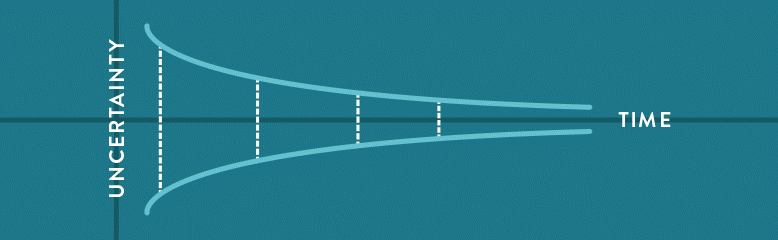
4. Data Outcomes Over Outputs
Making data a strategic organizational imperative should drive better outcomes. However, humans are not very good at dealing with uncertainty. We strive to control as many elements as possible, especially in business. This often leads to prioritizing outputs over outcomes, which undermines strategy implementation efforts.
Outputs are easy. Measuring outcomes is more challenging. Good data governance can help you stay focused on meeting target outcomes you hope to achieve versus a list of outputs which may or may not help you reach your goals.
- Define desired outcomes: Prioritize the outcomes you hope to achieve over the low-hanging fruit of outputs. A good theory of change process can help with this.
- Pivot if necessary: Don’t be afraid to abandon or rethink something that’s not working. Follow the data. Learn as you go. Stay lean.
5. Data Tools and Processes
Skills- and capacity-building is a common theme in this ten-point plan. Support data versatility within your organization by investing in tools and clear processes to help organizational stakeholders improve data science proficiency.
- Tools: Which data products and services are best for your organization? Vet these tools based on price point, features, benefits, and your team’s ability to get up to speed quickly. Be aspirational in the review process so your team can grow with these tools. However, don’t overextend yourselves either.
- Processes: Incorporate clear policies and procedures to drive data literacy throughout the organization. Workshop and co-create these to incorporate stakeholder input and get team buy-in.
6. Sustainable Data Infrastructure
Put flexible and secure systems in place for data storage, validation, and sharing. These will most likely be cloud-based services offered by third-party providers. Vet these providers and, if possible, prioritize vendors with clear policies:
- Security & data privacy: Current and emerging data privacy legislation requires that organizations protect stakeholder data. To reduce risk, find suppliers with clear cybersecurity and privacy policies.
- Data storage: Include sustainability principles, such as green hosting and data disposal when reviewing and vetting data storage options from suppliers.
- Scope 3 emissions: Related, find providers with clear sustainability commitments. Unfortunately, this can be difficult. However, it can also help you get ahead of the curve on tracking Scope 3 emissions, which are important to emerging climate disclosure and reporting legislation.
7. Predictive Analysis
Analytics providers increasingly include sophisticated machine learning models in their products and services. These tools recognize patterns found in organizational data and help people identify risks and opportunities. They are commonly used in auditing, forecasting, cash-flow prediction, and so on. Predictive analysis can also help marketers forecast potential campaign outcomes.
Consider the following when deciding whether predictive analytics tools are right for you:
- Qualify the need: Does your organization have enough data and the financial resources to make predictive analysis beneficial?
- Data privacy: When using these tools, will your data be used to train AI models? If so, does this put your organization at risk?
- Responsible analysis: Finally, with so many ethics and sustainability issues related to AI, how can you be sure your providers and your team are using these tools responsibly?

8. Data Equity & Risk
Data colonialism introduces new forms of inequality connected to the exploitation of data, which often replay and deepen those older inequalities, including racial and gender discrimination. And all of the developments with data extraction … are played out in a highly unequal global economy and geopolitics that have been profoundly shaped by colonialism.
— Data Grab: The New Colonialism of Big Tech and How to Fight Back, Ulises A. Mejias and Mick Couldry
Organizational risks related to data governance are on the rise. From fines and lawsuits to security breaches and data leaks, the data governance landscape is changing rapidly. This is especially true in regards to regulatory compliance.
To reduce risk, prioritize data equity across your business ecosystem when crafting data governance policies and practices, including:
- Internal stakeholders: Make access to data, digital tools, and training within the organization inclusive, equitable, and democratic.
- External stakeholders: Potential data and algorithmic biases are identified and removed. Third-party services are regularly audited for their privacy and security practices and other equity-related issues.
Below are some potential equity pitfalls to look out for:
- Equitable data standards: While we’re fans of using standards to drive meaningful change, data standardization has downsides as well. For example, standards tend to reflect the opinions and positions of those creating them. Often, this doesn’t represent an equitable cross-section of an organization’s data ecosystem, which can lead to unintended consequences.
- Algorithmic bias: Collect multiple stakeholder perspectives during strategy and design sessions to co-create solutions. This input can help reduce potential algorithmic bias. Also, ask third-party providers how they deal with algorithmic bias in their own products or services.
- Data exploitation: Finally, the extractive nature of modern data practices all but ensures some level of exploitation in an organization’s data value chain. If you use third-party data services for marketing, this might be hard to avoid. Consider due diligence processes to combat this. Prioritize moving to suppliers with clear risk reduction and data ethics policies.
While this is not legal advice, it is in any organization’s best interest to pay ongoing attention to legal and regulatory issues related to data governance and ethics. This is especially true for AI and other emerging technologies. Should you need legal advice, please consult a lawyer.
9. Nurture a Data Science Culture
Creating a workplace culture that prioritizes data science as a key organizational asset takes time and an ongoing commitment.
Consider the following:
- Governance ownership: Define who will lead and support your data science culture. This might be one person, a multidisciplinary or cross-departmental committee, or something that is shared from month-to-month. How will you build equity as a baseline into this culture?
- Safe to try: Striking the perfect balance between embracing new ideas and supporting existing data projects can be tough. Identify ‘safe to try’ experiments to help your organization innovate without unnecessarily draining its resources.
- Retrospectives: Similarly, schedule ongoing, regular retrospectives to share what was learned during each safe-to-try experiment. Adjust accordingly based on what you learn.
- Objectives-based training: Finally, ongoing training will be needed to improve team proficiency in advanced data science, especially as your ongoing experiments become more complex.

10. Staying in the Data Governance Game
Reflecting with intention is critical because whether you went to business school or beauty school, or were simply raised in Western culture, you have been steeped in a mindset that prioritizes planning and control over learning and adaptation.
— Pamela Meyer, Staying in the Game, p. 59
Finally, good data governance requires that we play the long game, regardless of setbacks, pitfalls, or unforeseen circumstances. Organizations must embrace uncertainty, adaptation, and continuous learning in order to create long-term success across data value chains.
By building what author Pamela Meyer calls Embodied Reflection and Action Cycles into ongoing team practices, you can learn and adapt as you go for continued success. According to Pamela, these cycles, based on agile processes, include four steps:
- What? Practicing Embodied Awareness and Assessment: This step invites you to become aware of what is going on with your whole embodied self and your environment.
- Gut? Engaging Your Whole Person: This step invites you to get in touch with what you are feeling in your heart and gut in response to what is happening.
- So What? Making Sense and Prioritizing: This step helps you become aware of what is happening and how to prioritize learning discoveries.
- Now What? Making Decisions and Acting: This step helps you review outcomes and facilitate a retrospective to prioritize action items and next steps.
While the processes outlined in Pamela’s book are geared toward individual leaders, their lessons apply to teams and disciplines as well. This is essential to good long-term data governance.
Sustainable Data Governance: Last Word
Rather than regarding data as a resource, we should understand it as human experience and social relations that are ‘datafied’ and thus transformed into a commodity, which then can be sold. This does not happen naturally, but requires a great deal of political intervention and violence and has grave consequences both for individuals and also for societies.
— Maximilian Jung, Digital Capitalism is a Mine not a Cloud, Transnational Institute
Data is a powerful ally in the quest to create better organizations and a better future overall. Also, our data policies and practices—or lack thereof—have consequences. We cannot treat data as a commodity nor can we ignore its impact on people and the planet. Yet, based on their business models, most tech companies need us to do just that.
Instead, let’s acknowledge that people drive governance policies and practices which sometimes lead to injustice, inequality, and undermining social structures. Let’s strive to create responsible data governance strategies that improve our organizations, respect human and non-human stakeholders, and drive sustainability efforts.
If you have questions about how to do this or want to share feedback on this ten-point data governance plan, please feel free to contact us.
Improve Your Data Privacy & Security Practices
Download our free Data Privacy Checklist
Download the Checklist